How ACOs Use Data Analytics to Improve Outcomes and Reduce Financial Risk
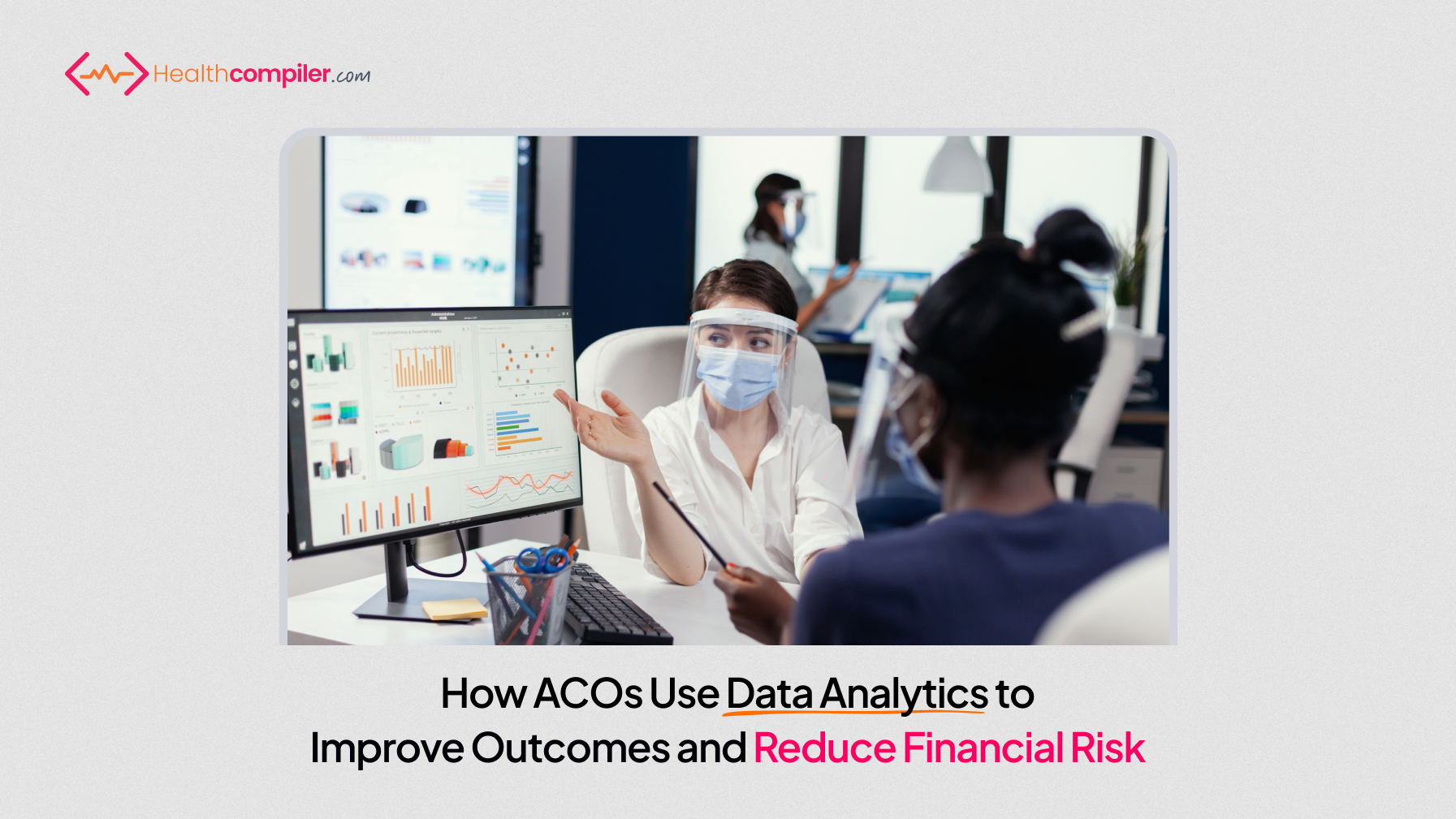
Accountable Care Organizations (ACOs) have become a cornerstone in the transformation of the healthcare industry. These organizations aim to improve patient outcomes while simultaneously reducing costs. A crucial tool in achieving these goals is data analytics. By leveraging data analytics, ACOs can enhance care coordination, identify high-risk patients, and implement preventative measures effectively. This blog explores how ACOs use data analytics to drive improvements in healthcare outcomes and reduce financial risk.
Understanding ACOs
An Accountable Care Organization is a network of doctors, hospitals, and other healthcare providers who voluntarily come together to provide coordinated high-quality care to their Medicare patients. The primary objective is to ensure that patients, especially those with chronic conditions, receive the right care at the right time, while avoiding unnecessary duplication of services and preventing medical errors. ACOs are incentivized to reduce costs and improve quality through shared savings programs.
The Role of Data Analytics in ACOs
1. Enhanced Care Coordination
One of the primary ways ACOs use data analytics is to enhance care coordination. By integrating and analyzing data from various sources, ACOs can create a comprehensive view of a patient's health history. This holistic view allows healthcare providers to:
- Track patient progress across different care settings.
- Identify gaps in care and address them proactively.
- Ensure that care plans are followed and adjusted as needed.
For instance, data analytics can highlight when a patient has missed a follow-up appointment or has not filled a prescription, enabling care teams to intervene promptly.
2. Identifying High-Risk Patients
Predictive analytics plays a pivotal role in identifying patients who are at high risk of developing chronic conditions or experiencing adverse health events. By analyzing historical data and identifying patterns, ACOs can:
- Predict which patients are likely to be readmitted to the hospital.
- Identify patients who are at risk of complications from chronic diseases.
- Implement targeted interventions to prevent hospitalizations and emergency room visits.
For example, predictive models can flag patients with diabetes who are at high risk of complications, allowing care teams to prioritize and customize their care plans.
3. Implementing Preventative Measures
Preventative care is a key strategy for improving patient outcomes and reducing costs. Data analytics helps ACOs to:
- Monitor population health trends and identify emerging health issues.
- Design and implement preventative care programs tailored to specific patient populations.
- Track the effectiveness of these programs and make necessary adjustments.
Through data analytics, ACOs can ensure that patients receive appropriate screenings, vaccinations, and other preventative services, ultimately reducing the incidence of severe health conditions and lowering healthcare costs.
4. Improving Clinical Decision-Making
Data analytics provides clinicians with actionable insights that improve decision-making at the point of care. By analyzing real-time data, clinicians can:
- Make evidence-based decisions that enhance patient care.
- Identify best practices and standardize care protocols across the organization.
- Reduce variability in care delivery and improve overall quality.
For example, clinical decision support systems powered by data analytics can alert physicians to potential drug interactions or recommend alternative treatments based on the latest research.
5. Reducing Financial Risk
By utilizing data analytics, ACOs can better manage financial risk through:
- Monitoring and controlling healthcare spending.
- Identifying areas where cost savings can be achieved without compromising quality.
- Participating in value-based payment models that reward efficiency and quality.
Data analytics helps ACOs track performance metrics, such as hospital readmission rates and patient satisfaction scores, which are often tied to financial incentives in value-based care models.
Case Study: Successful Implementation of Data Analytics in an ACO
A notable example of successful data analytics implementation is the Montefiore ACO in New York. Montefiore uses a robust data analytics platform to integrate data from various sources, including electronic health records (EHRs), claims data, and social determinants of health. This integrated approach has enabled Montefiore to:
- Reduce hospital readmissions by 30%.
- Achieve significant cost savings through targeted interventions.
- Improve patient outcomes by providing personalized care plans.
Conclusion
Data analytics is a powerful tool that enables ACOs to improve patient outcomes while reducing financial risk. By enhancing care coordination, identifying high-risk patients, implementing preventative measures, improving clinical decision-making, and managing financial risk, ACOs can achieve their goals of providing high-quality, cost-effective care. As the healthcare industry continues to evolve, the role of data analytics in ACOs will only become more critical in driving sustainable improvements in patient care and operational efficiency.